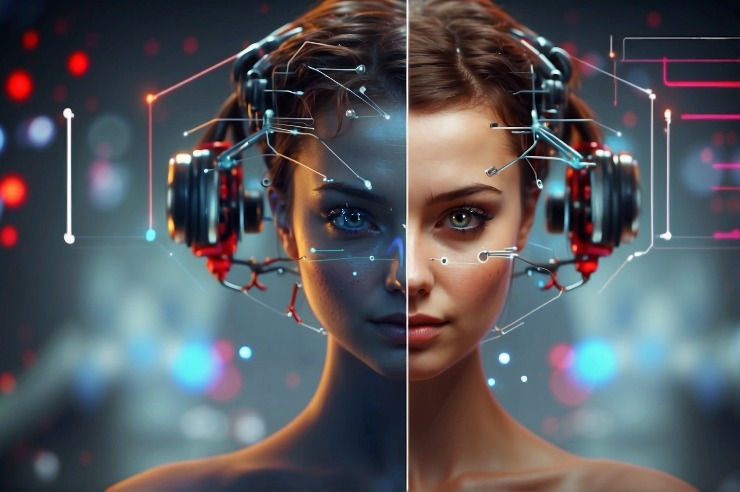
Understanding and Mitigating AI Hallucinations: Strategies for Reliable AI Systems
In the rapidly advancing field of artificial intelligence (AI), ensuring the reliability and accuracy of AI systems remains a critical challenge. One of the significant issues encountered in this realm is AI hallucinations—instances where artificial intelligence systems produce incorrect, misleading, or unintended outputs. These errors often stem from biases or errors present in the training data and can have serious implications for the effectiveness and trustworthiness of AI technologies. To address these challenges and reduce the likelihood of AI hallucinations, several strategies can be employed.
Defining AI Hallucinations:-
AI hallucinations refer to situations where an AI system generates outputs that are not grounded in reality, often producing responses that are inaccurate or nonsensical. These occurrences can arise from various sources, including biases in the training data, limitations in the model's design, or flaws in the algorithms used. Hallucinations can undermine the reliability of AI systems, making it crucial for developers and researchers to implement strategies that minimise their occurrence and impact.
Diverse and Representative Training Data:-
One of the most fundamental strategies to avoid AI hallucinations is to ensure that the training data used to develop the AI system is diverse and representative. Training data serves as the foundation for machine learning models, and if this data is biased or unrepresentative of real-world scenarios, the AI system is likely to produce skewed or inaccurate outputs.
To achieve this, developers should incorporate a wide range of examples into the training dataset, reflecting the variety of situations and contexts the AI system will encounter. This includes ensuring that data represents different demographics, geographical locations, and contextual variations. By doing so, the AI system can learn to generalise better and reduce the likelihood of producing biased or incorrect outputs.
Bias Detection and Mitigation:-
Bias in AI systems can significantly contribute to the occurrence of hallucinations. Therefore, regular assessment and mitigation of biases are essential steps in improving the reliability of AI models. Various tools and techniques are available to detect and address biases in machine learning models.
Developers can employ methods such as re-sampling, re-weighting, and using specialized algorithms designed to reduce bias in the training data. Additionally, implementing fairness-aware machine learning techniques can help identify and correct biases that may lead to hallucinations. By proactively addressing bias, developers can create more balanced and accurate AI systems.
Regular Updates and Retraining:-
AI models are not static; they require periodic updates and retraining to remain relevant and effective. As the environment and data evolve, so too should the AI systems. Regularly updating and retraining models with new and relevant data helps ensure that the AI system adapts to changes and remains accurate over time.
This process involves incorporating recent data into the training set, fine-tuning the model, and validating its performance. Regular updates help mitigate the risk of AI hallucinations by allowing the model to learn from new patterns and trends, reducing the likelihood of outdated or incorrect outputs.
Adversarial Testing:-
Adversarial testing is a valuable strategy for identifying vulnerabilities and weaknesses in AI systems. This approach involves designing intentional adversarial scenarios where inputs are crafted to mislead or challenge the model. By subjecting the AI system to such scenarios, developers can uncover potential weaknesses and areas where the model may be prone to hallucinations.
Adversarial testing helps in understanding how the AI system responds to unexpected or challenging inputs, allowing developers to make improvements and enhance the model's robustness. This proactive approach is crucial for strengthening the reliability of AI systems and reducing the risk of erroneous outputs.
Explainability and Transparency:-
Choosing AI models that are explainable and transparent is essential for understanding how the model arrives at its decisions. Explainability refers to the ability to interpret and understand the rationale behind an AI system's outputs. Transparent models provide insights into the decision-making process, making it easier to identify potential sources of hallucinations.
By selecting or designing AI models with built-in explainability features, developers can gain valuable insights into the inner workings of the system. This understanding allows for more effective debugging and refinement, ultimately leading to a reduction in hallucinations and an improvement in overall system reliability.
Human Oversight:-
Implementing human oversight in critical decision-making processes where AI is involved provides an additional layer of safety. Human intervention can help catch errors and prevent hallucinations from having significant consequences. This approach is particularly important in high-stakes applications, such as healthcare or finance, where the impact of AI errors can be substantial.
Human oversight involves having experts review and validate AI-generated outputs, providing an opportunity to correct inaccuracies before they affect real-world decisions. By combining human judgment with AI capabilities, organisations can enhance the reliability of their systems and mitigate the risk of hallucinations.
Ethical Guidelines and Standards:-
Adhering to ethical guidelines and standards is crucial when developing and deploying AI systems. Ethical considerations include evaluating the societal impact, fairness, and accountability of AI technologies. By integrating ethical principles into the development process, developers can address potential issues that may lead to hallucinations or biased outputs.
Establishing ethical guidelines helps ensure that AI systems are designed and implemented in a manner that prioritizes accuracy, fairness, and transparency. This proactive approach contributes to the creation of AI technologies that are both reliable and aligned with societal values.
User Feedback and Monitoring:-
Collecting feedback from users is an essential component of maintaining and improving AI systems. Users can provide valuable insights into potential issues and inaccuracies that may not be immediately apparent during development. Regularly monitoring the performance of AI systems and incorporating user feedback helps identify and address unintended consequences.
By establishing mechanisms for ongoing feedback and performance monitoring, developers can continuously refine their AI systems and address issues related to hallucinations. This iterative process ensures that the system remains responsive to real-world challenges and maintains high levels of accuracy.
Error Handling and Fail-Safes:-
Implementing robust error handling mechanisms and fail-safes is crucial for mitigating the impact of AI hallucinations. Error handling involves setting confidence thresholds for decision-making, where the system only acts on outputs that meet a certain level of confidence. Fail-safes include backup systems or alternative methods for handling situations where the AI system may produce unreliable outputs.
These strategies help minimize the impact of errors and ensure that the AI system operates safely and effectively, even in the presence of occasional inaccuracies. By incorporating error handling and fail-safes, developers can enhance the resilience of their AI systems and reduce the risk of adverse consequences.
Collaboration and Peer Review:-
Engaging in collaborative efforts and peer reviews within the AI community contributes to the development of best practices for avoiding hallucinations. Sharing knowledge and experiences with other researchers and practitioners helps identify common challenges and effective solutions.
Collaboration fosters a culture of continuous learning and improvement, enabling developers to stay informed about the latest advancements and methodologies in AI. Peer reviews provide an opportunity for external validation and feedback, further enhancing the reliability and accuracy of AI systems.
Conclusion:-
AI hallucinations represent a significant challenge in the development of reliable AI systems. By employing a combination of strategies, including diverse and representative training data, bias detection and mitigation, regular updates and retraining, adversarial testing, explainability, human oversight, ethical guidelines, user feedback, error handling, and collaboration, developers can work towards creating AI systems that are more accurate, transparent, and less prone to hallucinations.
As AI technology continues to advance, addressing these challenges will be crucial for ensuring the reliability and effectiveness of AI systems. By implementing these strategies, developers can enhance the robustness of their AI technologies and build systems that better serve users and meet the demands of real-world applications.
Decoding Legal Team
Comments